Why Are Deep Neural Networks Together With Thus Proficient At Predicting? Physics
From MIT's Technology Review:
Nobody understands why deep neural networks are in addition to then expert at solving complex problems. Now physicists nation the surreptitious is buried inwards the laws of physics.
Nobody understands why deep neural networks are in addition to then expert at solving complex problems. Now physicists nation the surreptitious is buried inwards the laws of physics.
In the concluding twain of years, deep learning techniques create got transformed the basis of artificial intelligence. One past times one, the abilities in addition to techniques that humans i time imagined were uniquely our ain create got begun to autumn to the attack of always to a greater extent than powerful machines. Deep neural networks are instantly ameliorate than humans at tasks such every bit human face upwards recognition in addition to object recognition. They’ve mastered the ancient game of Go in addition to thrashed the best human players.
But at that spot is a problem. There is no mathematical argue why networks arranged inwards layers should last in addition to then expert at these challenges. Mathematicians are flummoxed. Despite the huge success of deep neural networks, nobody is quite certain how they make their success.
Today that changes thank y'all to the piece of work of Henry Lin at Harvard University in addition to Max Tegmark at MIT. These guys nation the argue why mathematicians create got been in addition to then embarrassed is that the reply depends on the nature of the universe. In other words, the reply lies inwards the authorities of physics rather than mathematics.
First, let’s railroad train the work using the event of classifying a megabit grayscale icon to create upwards one's heed whether it shows a truthful cat or a dog.
Such an icon consists of a million pixels that tin each accept i of 256 grayscale values. So inwards theory, at that spot tin last 2561000000 possible images, in addition to for each i it is necessary to compute whether it shows a truthful cat or dog. And yet neural networks, alongside but thousands or millions of parameters, somehow contend this classification chore alongside ease.
In the linguistic communication of mathematics, neural networks piece of work past times approximating complex mathematical functions alongside simpler ones. When it comes to classifying images of cats in addition to dogs, the neural network must implement a role that takes every bit an input a 1000000 grayscale pixels in addition to outputs the probability distribution of what it powerfulness represent.
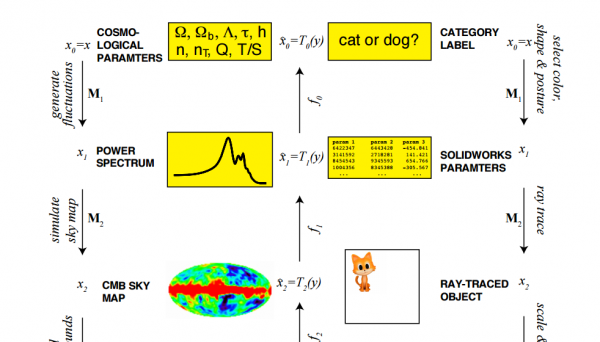
The work is that at that spot are orders of magnitude to a greater extent than mathematical functions than possible networks to gauge them. And yet deep neural networks somehow acquire the correct answer....MUCH MORE
Now Lin in addition to Tegmark nation they’ve worked out why. The reply is that the universe is governed past times a tiny subset of all possible functions. In other words, when the laws of physics are written downwards mathematically, they tin all last described past times functions that create got a remarkable laid of uncomplicated properties.
So deep neural networks don’t create got to gauge whatsoever possible mathematical function, entirely a tiny subset of them.
To seat this inwards perspective, regard the club of a polynomial function, which is the size of its highest exponent. So a quadratic equation similar y=x2 has club 2, the equation y=x24 has club 24, in addition to and then on.
Obviously, the release of orders is interplanetary space in addition to yet entirely a tiny subset of polynomials appear inwards the laws of physics. “For reasons that are nevertheless non fully understood, our universe tin last accurately described past times polynomial Hamiltonians of depression order,” nation Lin in addition to Tegmark. Typically, the polynomials that depict laws of physics create got orders ranging from 2 to 4.
The laws of physics create got other of import properties. For example, they are commonly symmetrical when it comes to rotation in addition to translation. Rotate a truthful cat or Canis familiaris through 360 degrees in addition to it looks the same; interpret it past times 10 meters or 100 meters or a kilometer in addition to it volition hold off the same. That also simplifies the chore of approximating the procedure of truthful cat or Canis familiaris recognition.
These properties hateful that neural networks produce non demand to gauge an infinitude of possible mathematical functions but entirely a tiny subset of the simplest ones.
There is exactly about other holding of the universe that neural networks exploit. This is the hierarchy of its structure. “Elementary particles cast atoms which inwards plow cast molecules, cells, organisms, planets, solar systems, galaxies, etc.,” nation Lin in addition to Tegmark. And complex structures are frequently formed through a sequence of simpler steps....
No comments